Data integrity assurance is crucial in today’s digital world, as it underpins decision-making and organizational operations. Compromised data integrity can lead to serious consequences. Poor decision-making can occur when decisions based on incorrect or manipulated data lead to operational, financial, or strategic issues. Businesses, especially in sectors like banking, healthcare, and e-commerce, risk losing customer trust if data isn’t kept accurate and secure. Violating compliance with standards like GDPR, HIPAA, and PCI-DSS due to data integrity breaches can result in fines, legal problems, and repetitional damage. Inaccurate data can disrupt operations, cause security breaches, lead to financial losses, and damage an organization’s reputation. These risks highlight the importance of maintaining data integrity for the security and success of any organization.
How data Is Compromised?
Data can lose its integrity through several ways, including bad data collection practices which lead to inaccurate or incomplete data being captured. When data is stored in multiple locations, parts of it can be lost or deleted, leading to inconsistencies. Mishandling or mis-coding of data during processing or transfer can also corrupt it. Furthermore, data containing unsafe or inappropriate words can compromise its quality and usability. Lastly, data aggregated from various sources, like third-party vendors, internal systems, human input, or different programming languages, can lead to discrepancies and inaccuracies if not properly integrated and validated. These factors collectively contribute to the degradation of data integrity.
Compromised Data Lead to Failure
In here I want us to take a look at a startup company located in South America that relay on data from a 3rd party vendor for it’s success and here we can see what happens when data is compromised. Bus4Me(name is hidden), a tech startup, launched a public transportation app using data from multiple sources, including third-party vendors. However, they faced a challenge when outdated data from a vendor led to the app displaying incorrect bus schedules and locations. This caused significant disruptions for commuters, resulting in missed buses and user dissatisfaction.
Many users uninstalled the app and shared negative feedback, damaging the app’s reputation. Upon identifying the outdated data as the root cause, Bus4Me suspended its use, apologized publicly, and revamped its data validation processes, including a new feature for user-reported discrepancies. The incident highlighted the importance of data accuracy and robust validation, especially with third-party data, and although Bus4Me recovered, it experienced a temporary loss of trust and reputation. Bus4Me is a great example how relaying that data is simply trusted, and in this case it’s not as simple as it looks like.
Whats ALCOA+?
As part of Data Integrity Assurance we look for security, and ISMS frameworks to maintain and protect our data integrity and in here we’ll take a look at a recommended secure framework that we can provide to our team and relevant stockholders in our organization so they will follow and ensure that data is of highest quality.
- Attributable: Data must clearly show who collected or generated it, its source, and when it was captured. This ensures accountability and traceability. Traceability will allow to understand whether if the data is sourced correctly or had an issues through the data pipe line.
- Risk: Shared passwords can make data unattributable.
- Eurotherm Remedy: Implements password-protected accounts with role-defined permissions, logging actions in an audit trail and integrating with systems like Microsoft® Active Directory.
- Legible: Data must be readable and permanent, understandable years after recording. Meaning that data can’t have minor errors, or usage of terms that are trends(or at least those need to be covered and explained).
- Risk: Paper records are prone to alteration and scaling errors.
- Solutions: Digital availability of process data and alarm history in readable formats through various software and interfaces.
- Contemporaneous: Data should be recorded at the time of the activity. The date of the data may change the context of the data, hence its important to understand when data created, when it was published when data recorded and much more different timestamps and type of timestamps.
- Risk: Lack of timestamping can lead to backdating.
- Solutions: Automatic recording of process data and metadata with timestamps.
- Original: Data should be in its unaltered state or a ‘true copy’. it’s easy to apply mall fixes to data, however doing so might compromise the original copy of the data which might lead to a completely different meaning by context of the data.
- Risk: Paper and easily editable digital records can be modified.
- Solutions: Data recorded and stored in tamper-resistant formats.
- Accurate: Data must represent true values or actions. Different data source may or may not be accurate, From it’s type of data, the context or the simple “facts” of the data. easy example would be dating customer birthday incorrectly which leads to a (small) trust issue.
- Risk: Handwritten records and equipment inaccuracies can lead to errors.
- Solutions: Digital recording with high accuracy inputs and sensor positioning, alongside calibration and validation procedures.
- Complete (+): All information must be available and intact. Data that is partial or saved in portion in different locations might lead to a complete different result or expectations by anyone who uses the data.
- Risk: Recording in different places and communication dropouts can result in data loss.
- Solutions: Consolidated recording of process data and operator actions, with features to enhance data capture reliability.
- Consistent: Data should be chronologically coherent. timely manner data may effect the meaning of the data and previously recorded data might effect on future recorded data.
- Risk: Without timestamps, data sequence can’t be verified.
- Solutions: Chronological data recording with proper timestamping.
- Enduring: Data must be securely stored for long-term use. How we record the data can impact the longevity of the data. If we store data as paper or if we store data in a service that have TTL.
- Risk: Paper records can get lost or deteriorate. converting data type record from one to an other might result in compromised data (e.g paper to digital)
- Solutions: Long-term compatible digital recording formats for data preservation.
- Available: Data should be easily and quickly accessible. How and where we store data can impact it’s availability. If we store paper records or if we store data in a physical store or heavily guarded or behind a lot of security barriers it might not be as available as we think.
- Risk: Retrieving paper records or from multiple archives is slow.
- Solutions: High availability system architecture for quick data retrieval.
Summary
Maintain data is not a simple as it sound, and failure of data integrity can lead to dire consequences and in some cases to a failing business. We can’t simply trust data that we receive, no matter what the source. Data can’t be used “as is” there are principles and rules that need to be followed to the teeth so the quality of the data can be used in our organization so decisions be safely made and customers can trust us as a reliable source of information.
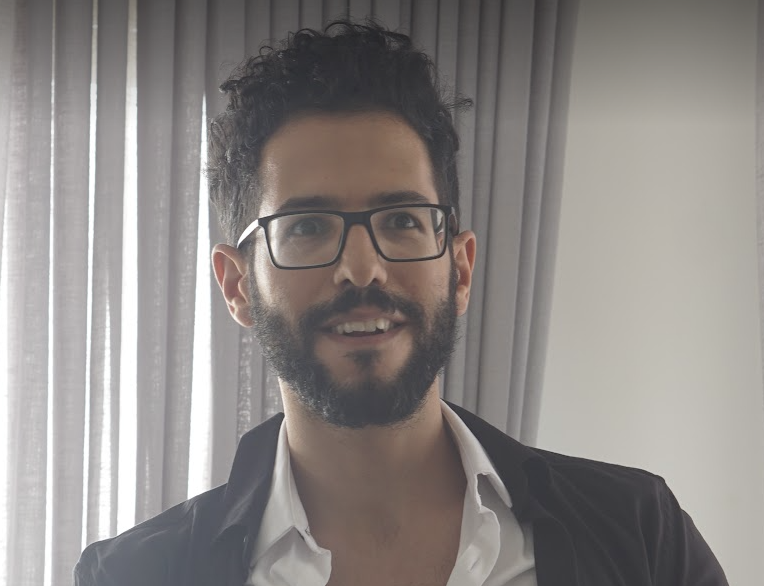
I am a software engineer with 20 years of experience of writing code, Software languages, Large scale web application, security and data protection of online digital assets in various software systems and services. I’ve decided to write and share my interests in cyber security online and information security to help and improve white hat security, safety and privacy of our online digital assets, As companies, as individuals or experts providing services. In here you’ll be able to read freely about cyber security threats, detections, common problems, services, news and everything related to information security and cyber security – enjoy and feel free to contact me via the contact page for any question.